Pitfalls to Predicting Patient Acuity
For over a decade, ClinIntell has developed advanced algorithms to accurately predict patient acuity at the system, hospital, service line, and physician levels. These predictions are tailored to reflect the unique characteristics of each hospital and the patient population served, recognizing that some facilities care for more complex patient populations than others. For example, patients in rural areas with serious medical conditions and/or multiple comorbidities and complications may travel long distances to urban academic medical centers. Factors such as bed size and resident-to-bed ratios play a role in predicting the acuity of the populations these hospitals serve.
Using Machine Learning to Identify Key Correlations
ClinIntell uses advanced machine learning algorithms to identify hospital demographics that correlate to patient acuity, particularly under the MS-DRG reimbursement system. These insights are validated across hospitals not included in the model development, ensuring they are generalizable. This approach has also improved the accuracy of predictions when applied to unrelated claims from ClinIntell’s clients.
Analyzing Variables That Impact Patient Acuity
To refine its models, ClinIntell has analyzed hundreds, if not thousands, of variables from trusted sources such as CMS, Definitive Healthcare, the CDC, and the U.S. Census Bureau. The value of a variable depends on its relationship with CC/MCC severity. For example, while bed size often correlates with higher severity, the effect diminishes for larger hospitals. In such cases, using transformed variables—like raising bed size to the third power—provides better predictive accuracy.
There may be more complicated ways to construct synthetic variables. For example, a new bed size variable can be created based on the hospital’s IPPS status and if it is in a rural versus an urban environment. By leveraging machine learning libraries in R to process tens of millions of claims, ClinIntell identifies the most meaningful variables with the strongest correlations.
The Challenge: Patient Severity vs. Hospital Resourcing
Years of analysis have revealed that documented patient severity often reflects hospital resources more than the actual health status of the patient population. For example, ClinIntell assessed 74 variables from the CDC, along with over 100 Census Bureau measures, as potential predictors of hospital-level CC/MCC severity. Out of these, 39 variables demonstrated some predictive value. The top 10 variables accounted for two-thirds of the total weight in the model, but many produced unexpected results. For instance:
- Hospitals in areas with higher binge drinking rates were associated with lower CC/MCC severity, which seems counterintuitive.
- Obesity rates in the hospital’s community showed a negative correlation with CC/MCC severity, even though higher obesity rates are typically linked to poorer health outcomes.
These findings suggest that such variables might reflect the socio-economic conditions of the community served rather than actual health risks. Key observations include:
- Metropolitan population size (variable #2): Likely reflects the wealth of larger cities rather than directly influencing severity.
- Education levels (variable #9): May indicate socio-economic status, which correlates to hospital resources.
- Depression diagnoses: It is unclear if this variable reflects higher complication risks or simply correlates with socio-economic factors.
Ultimately, these findings suggest that documentation and coding accuracy are strongly influenced by hospital resources, such as CDI staffing, technology, and consulting—factors often linked to the community's socio-economic status.
%2520(1).png)
The Solution
ClinIntell has evaluated hundreds of variables from various governmental and industry data sources to build its predictive models. However, only a small subset of these variables, along with synthetic variables derived from them, are ultimately included in ClinIntell’s algorithms. This selective approach ensures the models focus on the most meaningful and reliable predictors while avoiding variables that may introduce bias or misinterpretation.
Concerning Conclusions
Outcome measures, in most cases, need to be risk-adjusted to account for the population being analyzed. Hospitals serving sicker patients with multiple acute conditions are expected to have higher mortality rates. However, if risk adjustment models are skewed by hospital resources that affect documentation and coding, quality assessments may be artificially high. This highlights the importance of considering both population health and hospital resourcing when evaluating outcomes.
Case Mix Index Analysis – Free Personalized Demo
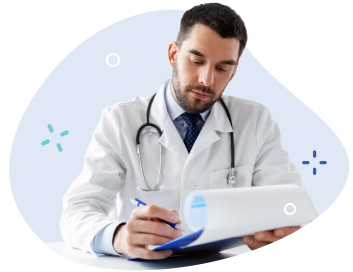